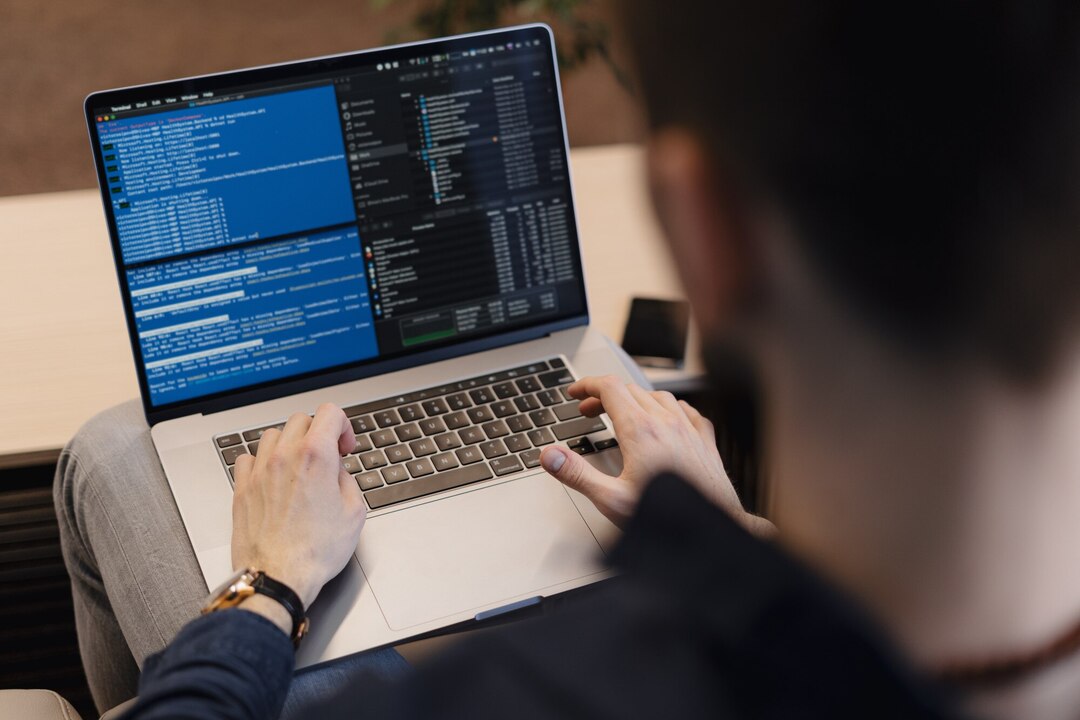
The energy market is in constant flux, influenced by factors like demand, supply, weather conditions, and geopolitical events. As prices swing up and down, the need for accurate predictions becomes crucial. Enter artificial intelligence—an innovative force reshaping how we approach energy price forecasting. With its ability to analyze vast amounts of data at lightning speed, AI offers insights that were once unimaginable.
Imagine a world where energy companies can anticipate price shifts with remarkable precision. This isn’t just a dream; it’s becoming reality thanks to AI-powered solutions. From optimizing resource allocation to enhancing risk management strategies, these technologies are transforming markets across the globe.
Join us as we explore how AI is revolutionizing energy price forecasting and what it means for businesses and consumers alike. The future of energy pricing might just be smarter than you think!
How AI is Revolutionizing Energy Market Predictions
Artificial intelligence is transforming the landscape of energy market predictions. Traditional methods often rely on historical data and simplistic models, which can miss critical nuances. AI brings advanced algorithms that analyze vast amounts of data in real-time.
These intelligent systems can identify patterns and correlations that human analysts might overlook. Machine learning techniques adapt as new information emerges, continuously improving accuracy. This responsiveness allows for timely adjustments in strategies based on shifting market dynamics.
Moreover, AI tools integrate diverse datasets—like weather forecasts, economic indicators, and consumer behavior trends—to create more comprehensive models. The result is a clearer picture of future energy prices.
As these technologies evolve, they empower companies to make informed decisions faster than ever before. With greater precision comes enhanced risk management capabilities and optimized resource allocation across the board.
Benefits of AI in Energy Price Forecasting
AI brings remarkable advantages to energy price forecasting. One significant benefit is enhanced accuracy. Machine learning algorithms analyze vast datasets, identifying patterns that human analysts might miss.
Increased speed is another key advantage. AI processes data in real-time, allowing companies to react quickly to market changes and adjust strategies accordingly.
Cost efficiency also improves with AI integration. Automated systems reduce manpower needs while delivering consistent results. This allows organizations to allocate resources more effectively.
Moreover, predictive analytics help businesses anticipate demand fluctuations better than ever before. Understanding these trends can lead to smarter procurement strategies and reduced risks.
AI fosters innovation in modeling techniques, enabling the exploration of new variables impacting prices. This flexibility opens doors for advanced simulation scenarios and improved decision-making frameworks across the industry.
Case Studies: Successful Implementation of AI in Energy Companies
One notable case study is that of a leading European utility company. By implementing AI algorithms, they transformed their pricing models and improved forecasting accuracy by over 30%. This change not only enhanced decision-making but also optimized resource allocation.
In another instance, an American energy firm utilized machine learning to analyze historical data patterns. The results were impressive; predictive analytics reduced operational costs significantly while maximizing profit margins on energy sales.
A pioneering startup focused on renewable sources adopted AI for real-time price adjustments based on market demand fluctuations. Their innovative approach led to better customer satisfaction and increased competitiveness in the marketplace.
These examples highlight how diverse companies leverage AI technologies, carving out new pathways for efficiency and profitability in the ever-evolving energy sector. Each success story underscores the transformative potential of artificial intelligence within the industry landscape.
Challenges and Limitations of AI-Powered Energy Price Forecasting
Despite the benefits, AI-powered energy price forecasting faces several challenges. Data quality is a significant issue. Inaccurate or incomplete data can skew predictions and lead to poor decisions.
Additionally, the complexity of energy markets poses hurdles. Factors like geopolitical events, supply chain disruptions, and regulatory changes introduce unpredictability that algorithms might not fully capture.
Moreover, there’s a risk of over-reliance on technology. While AI excels in pattern recognition, it may overlook nuanced human insights and market sentiment that experienced analysts provide.
Training models requires substantial resources and expertise. Many companies struggle with staffing skilled personnel who understand both AI techniques and energy markets.
Ethical considerations arise when using sensitive customer data for training AI systems. Balancing innovation with privacy concerns remains a delicate task for organizations venturing into this space.
Future Outlook and Potential Impact on the Energy Industry
The future of AI-powered energy price forecasting looks promising. As algorithms become more sophisticated, they will harness vast amounts of data with greater precision. This shift could lead to unprecedented accuracy in predicting market trends.
Energy companies may find themselves empowered by these insights. With improved forecasts, they can better manage supply chains and optimize pricing strategies. This agility could transform how businesses respond to fluctuations in demand and shifting regulatory landscapes.
Furthermore, the integration of AI tools may foster collaboration among industry players. Shared insights on predictive models can enhance collective understanding of market dynamics.
As innovation continues, we might also see a rise in decentralized energy systems powered by real-time data analysis. These advancements have the potential to reshape traditional business models within the sector entirely, paving the way for a more resilient energy ecosystem.
Conclusion
AI-powered solutions are transforming the landscape of energy price forecasting. As the industry continues to evolve, embracing advanced technologies like machine learning and big data analytics becomes essential for staying competitive. The benefits are clear: enhanced accuracy in predictions, better risk management, and improved operational efficiencies.
Successful case studies demonstrate that companies leveraging AI can forecast fluctuations with remarkable precision. However, challenges such as data quality and integration issues still exist. Navigating these hurdles will be crucial for maximizing AI’s potential in energy markets.
Looking ahead, the future of AI in energy price forecasting appears bright. Continued advancements promise to refine predictive models further, enabling companies to make informed decisions that drive profitability and sustainability. As we move toward a more digitalized world, those who adapt swiftly will likely lead the charge into an innovative era within the energy sector.