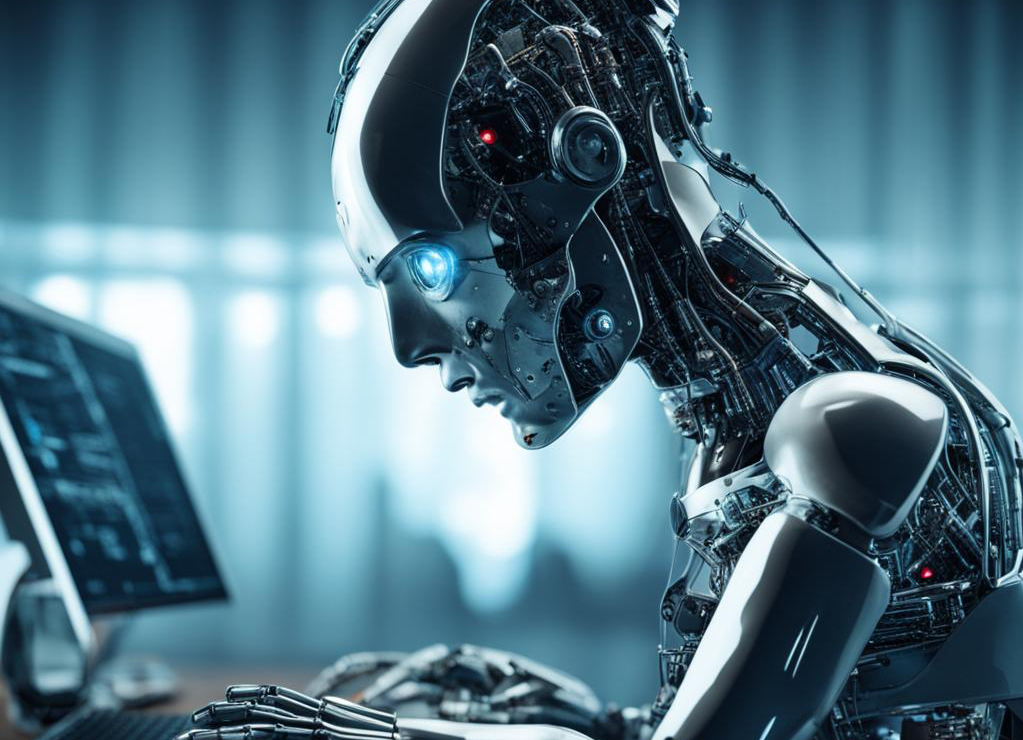
Welcome to the age of artificial intelligence (AI) and its transformative impact on asset management! In this fast-paced digital world, organizations are constantly seeking innovative ways to optimize their operations and maximize returns. And AI is emerging as a game-changer in the field of asset management. By leveraging advanced algorithms and machine learning capabilities, AI enables businesses to make data-driven decisions, enhance efficiency, and improve overall portfolio performance.
We will explore the power of AI-driven strategies for effective asset management. We’ll delve into the benefits that AI brings to the table, examine real-world use cases where AI has proven its worth in optimizing assets, discuss challenges and limitations to be aware of, highlight best practices for implementing AI in asset management processes, and finally peek into what lies ahead for the future evolution of this exciting technology.
Benefits of AI in Asset Management
AI has revolutionized the field of asset management, bringing numerous benefits to both individuals and organizations. One key advantage is enhanced decision-making capabilities. With AI-powered algorithms analyzing vast amounts of data, asset managers can make more informed and accurate investment decisions.
Another benefit is improved efficiency and cost-effectiveness. AI automates repetitive tasks such as data entry and analysis, allowing asset managers to focus on higher-value activities. This not only saves time but also reduces operational costs.
AI enables proactive risk management by identifying potential risks and predicting market trends. By leveraging machine learning models, asset managers can mitigate risks before they escalate and seize opportunities for growth.
AI enhances portfolio optimization by providing real-time insights into market conditions and customer preferences. This allows for the creation of customized investment strategies tailored to individual needs.
AI-driven chatbots have transformed client interactions by providing instant assistance and personalized recommendations based on user behavior patterns.
The integration of AI in asset management yields significant benefits such as improved decision-making capabilities, increased efficiency, proactive risk management, enhanced portfolio optimization, and superior client engagement.
Use Cases of AI in Asset Management
AI has revolutionized the field of asset management, offering numerous use cases that enhance efficiency and improve decision-making. Let’s explore some of these applications.
- Predictive Maintenance: AI-driven algorithms can analyze historical data and real-time sensor inputs to predict equipment failure or maintenance needs. This helps prevent costly downtime and optimize maintenance schedules.
- Portfolio Optimization: AI-powered algorithms can analyze vast amounts of data to construct optimized investment portfolios based on factors like risk tolerance, market trends, and performance indicators. This enhances portfolio diversification and maximizes returns.
- Fraud Detection: By analyzing large volumes of financial transactions, AI systems can identify patterns indicative of fraudulent activities. This enables timely detection and prevention of fraud while minimizing losses.
- Risk Assessment: AI models can assess market risks by analyzing various factors such as economic conditions, geopolitical events, and industry-specific information. It provides insights into potential risks that may impact asset performance.
- Sentiment Analysis: Using natural language processing techniques, AI can analyze news articles, social media posts, and customer reviews to gauge investor sentiment towards specific assets or companies. This helps in making informed investment decisions.
- Intelligent Chatbots: Asset management firms are leveraging AI-powered chatbots to provide personalized assistance and support to clients at any time without the need for human intervention.
The use cases mentioned above are just a glimpse into the possibilities offered by integrating artificial intelligence into asset management processes. By utilizing these technologies effectively, firms can gain a competitive edge by improving operational efficiency, making smarter investment decisions, and mitigating risks effectively.
Challenges and Limitations of AI in Asset Management
Implementing AI in asset management certainly brings numerous benefits, but it also comes with its fair share of challenges and limitations. One significant challenge is the need for accurate data. AI algorithms rely on large volumes of high-quality data to make informed decisions. However, gathering, cleaning, and organizing this data can be a time-consuming and complex process.
Another challenge is the potential for bias in AI models. If the training data used to develop an AI system contains biased information or reflects past discriminatory practices, then these biases may be perpetuated by the algorithm during decision-making processes.
Transparency can be a limitation when using AI in asset management. Some advanced machine learning algorithms are considered “black boxes,” meaning they are difficult to interpret or explain their reasoning behind specific recommendations or decisions.
There can be resistance from employees towards adopting new technologies like AI in asset management systems. This resistance might stem from concerns about job security or a lack of understanding about how these technologies will enhance their work rather than replace them.
Cybersecurity threats pose another challenge when leveraging AI in asset management systems. As organizations become increasingly reliant on technology-driven solutions powered by artificial intelligence, they must also remain vigilant against cyber attacks that could compromise sensitive financial information or disrupt critical operations.
Despite these challenges and limitations, organizations can overcome them through proactive measures such as investing in robust data collection processes, ensuring diversity and inclusivity while developing algorithms, promoting explainable AI approaches where possible, offering comprehensive employee training programs to foster technological literacy and cybersecurity awareness among staff members.
Best Practices for Implementing AI in Asset Management
Implementing AI in asset management can be a game-changer for organizations looking to optimize their operations and improve decision-making. However, it’s important to approach the implementation process with careful planning and consideration. Here are some best practices to keep in mind when incorporating AI into your asset management strategy.
Clearly define your objectives and identify the specific areas where you believe AI can provide value. Whether it’s predictive maintenance, risk assessment, or portfolio optimization, having a clear understanding of your goals will help guide the implementation process.
Ensure that you have access to high-quality data. AI algorithms rely heavily on data inputs for accurate predictions and insights. Therefore, it is essential to have clean, reliable data sets that adequately represent the assets you are managing.
Collaborate with domain experts throughout the implementation process. While AI technologies can offer valuable insights and automation capabilities, they should not replace human expertise entirely. By involving subject matter experts in the development and training of AI models, you can enhance their accuracy and relevance.
Prioritize explainability and transparency in your AI systems. As asset managers make critical decisions based on AI-generated insights, it is crucial to understand how those conclusions were reached. Implementing techniques like interpretable machine learning algorithms or model-agnostic methods can help shed light on the reasoning behind recommendations made by these systems.
Continuously monitor and evaluate your AI models’ performance over time. Regularly updating models based on changing conditions or new data ensures that they remain effective tools for decision-making within asset management processes.
By following these best practices during an AI implementation journey within asset management operations allows businesses to unlock its full potential while mitigating risks associated with adoption challenges effectively!
Future Outlook: The Role of AI in the Evolution of Asset Management
Artificial Intelligence (AI) has already made significant strides in transforming various industries, and asset management is no exception. As technology continues to advance at a rapid pace, the future outlook for AI in asset management looks promising.
One key area where AI is expected to play a crucial role is data analysis. With large volumes of data being generated every day, traditional methods of analyzing and interpreting this information can be time-consuming and prone to human error. AI-powered algorithms have the potential to analyze vast amounts of data quickly and accurately, providing valuable insights that can inform investment decisions.
Another aspect where AI holds great promise is risk management. By leveraging machine learning algorithms, asset managers can identify patterns and trends that might not be apparent to humans alone. This enables them to proactively manage risks by predicting market fluctuations or identifying potential investment opportunities.
AI-driven automation has the potential to streamline operational processes within asset management firms. Tasks such as portfolio rebalancing, trade execution, and reporting can be automated using intelligent algorithms, freeing up valuable time for asset managers to focus on strategic decision-making.
It’s important to acknowledge that there are challenges associated with implementing AI in asset management. One such challenge is ensuring transparency and accountability when relying on algorithmic decision-making processes. It becomes essential for firms to establish robust governance frameworks that address ethical concerns and regulatory compliance.
There may also be limitations regarding access to high-quality data needed for training AI models effectively. Data privacy concerns must also be addressed when dealing with sensitive client information.
Conclusion
As technology continues to advance at an unprecedented rate, AI-driven strategies are transforming the field of asset management. The benefits of incorporating AI into asset management practices are numerous and include improved efficiency, increased accuracy in decision-making, enhanced risk assessment capabilities, and cost savings.
The use cases of AI in asset management span across various industries such as finance, manufacturing, healthcare, and transportation. From predictive maintenance and anomaly detection to portfolio optimization and fraud detection, AI algorithms have proven their ability to analyze vast amounts of data quickly and provide actionable insights.
It is important to acknowledge that there are challenges and limitations associated with implementing AI in asset management. These include data quality issues, the need for skilled professionals to manage AI systems effectively, ethical considerations surrounding privacy and bias concerns.
To overcome these challenges and reap the full benefits of AI in asset management implementation best practices should be followed. This includes ensuring high-quality data collection processes; establishing a clear governance framework; integrating human expertise with AI technologies; continuously monitoring performance metrics; fostering transparent communication between stakeholders.
Looking ahead, the future outlook for AI in asset management appears promising. As machine learning algorithms continue to evolve through advancements like deep learning techniques or quantum computing applications. We can expect even more sophisticated approaches that will further enhance decision-making processes within this sector.
With its ability to process large volumes of information quickly,and make predictions based on complex patterns,it has become an indispensable tool for improving efficiency,reducing costs,and mitigating risks.
Incorporating best practices while being mindful of potential limitations will pave the way toward successful implementation.